Prof. LONG Qian from Yunnan Observatories of the Chinese Academy of Sciences, collaborating with Professor ER Xinzhong, the leader of this research, from cosmology group at SWIFAR of Yunnan University, identified 38 new strong lensing candidates by deep learning technique. The research was recently published online in Monthly Notices of the Royal Astronomical Society.
Galaxy-scale strong gravitational lensing systems serve as one of the most important probes to study a variety of problems in cosmology and astrophysics. For example, it allows researchers to probe the dark matter halos of galaxies, study the formation and evolution of galaxies, as well as estimate the Hubble constant.
However, the currently very limited samples of confirmed strong lenses have become the major bottleneck of such studies. With data from the next generation surveys, researchers expect to find tens of thousands strong lensing systems. How can people identify robustly and efficiently strong lenses from the huge amount of imaging data?
The development of Convolutional Neural Networks (CNNs) has made rapid progress in recent years, and this technique has been successfully applied to a variety of astronomical problems. In this study, researchers constructed a new CNN trained by simulated images of strong lensing systems.
With this network, they had identified 38 new strong lensing candidates in the KiDS (Kilo-Degree Survey) data collected with the European Southern Observatory (ESO) 2.6 meter VLT Survey Telescope (VST).
This study employed the new scientific computing language Julia to construct a fully customized deep neural network, which is designed to fit the intrinsic characteristics of the gravitational lens data and trained in a special way to increase the generalization ability.
Although the network is restricted to a small scale to suppress the over-fitting phenomenon, experiments proved that the accuracy of the obtained network is similar to large-scale networks. The network can work smoothly on ordinary PCs with or without GPU.
These features will help this new network becomes a good tool for astronomers, because it is relatively easy for them to train, test, and modify the network in real-time.
"The deep learning technique used in this research has the potential to be applied to tons of data of other sky surveys,” said Prof. LONG, one of the co-corresponding authors and also an expert in artificial intelligence area.
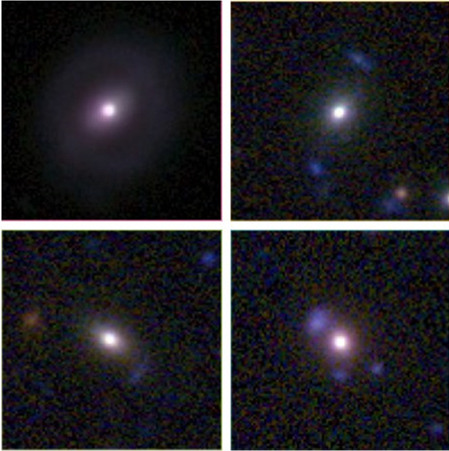
Images of 4 out of the 38 newly identified strong lensing candidates (credit: KiDs)
Contact:
LONG Qian, YNAO, CAS
longqian@ynao.ac.cn