Recently, Dr. DING Xu and Researcher JI Kaifan from Yunnan Observatories, the Chinese Academy of Sciences(CAS) utilized machine learning methods to search for contact binary candidates in the Transiting Exoplanet Survey Satellite (TESS) survey. This study was published in The Astronomical Journal.
Contact binaries are strongly interacting stellar systems where both stars fill their Roche lobes and share a common envelope. These systems are significant as potential progenitors of red novae, which can lead to stellar mergers, and typically have orbital periods of around 0.2 days. Given the importance of studying such short-period contact binaries, and the vast amounts of photometric data now available from large-scale surveys, there is a significant need for efficient methods to identify these objects.
To achieve this, the researchers implemented an unsupervised autoencoder neural network, which bypasses the need for manually labelled training data, thereby reducing labour costs. The autoencoder was trained to recognize contact binary light curves by measuring the reconstruction error between the input data and its own reconstruction. When the model was applied to other types of variable stars, an increase in reconstruction error indicated a deviation from the contact binary light curves, thereby identifying other variable star types.
The researchers utilized the Phoebe program to generate theoretical light curves based on contact binaries' parameters, and added noise to match the light curves observed by TESS. The model showed excellent performance in reconstructing contact binary light curves, with a global goodness of fit (R2) around 0.999. A threshold of 0.99 for global goodness of fit (R2) and 1.2 days for the period was set to filter out obvious outliers.
Additional constraints included range magnitude and local goodness of fit (R2). The researchers prioritized targets with a range magnitude greater than 0.1, as lower values often correspond to other variable star types, such as ellipsoidal or pulsating variables. A sliding window approach was used to calculate local goodness of fit (R2), further refining the selection of contact binary candidates. This method excluded targets with poor local goodness of fit (R2), resulting in a final dataset of 1,322 contact binary candidates.
This research was supported by the National Natural Science Foundation of China and the Special Scientific Research Fund for Astronomical Telescope of the China Manned Space Project.
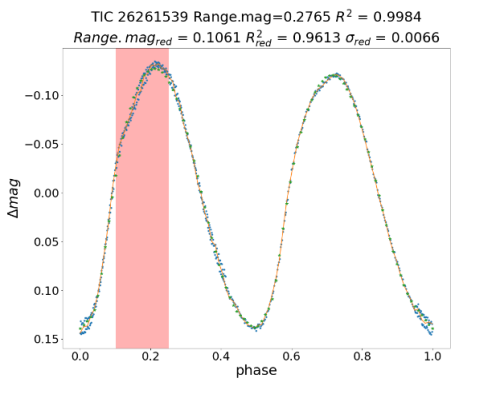
Figure 1, global and local goodness of fit (R2) calculations are performed using the reconstructed light curves to exclude RRc variables. Image by DING.
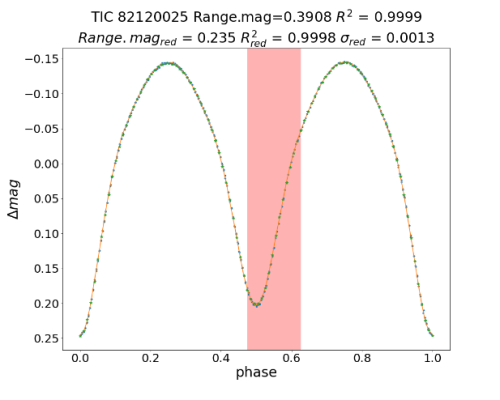
Figure 2, global and local goodness of fit (R2) calculations are performed using the reconstructed light curves to select contact binary candidates. Image by DING.
Contact:
DING Xu
Yunnan Observatories, CAS
E-mail:dingxu@ynao.ac.cn